Dive into the AI Dilemma: a problem facing data analysts and businesses owners alike.
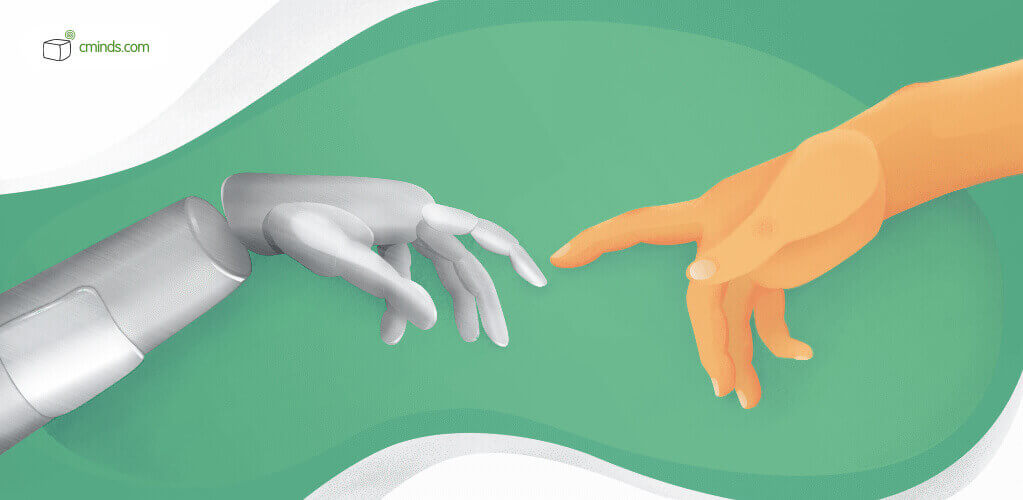
Artificial intelligence (A.I.) systems have prompted an exponential growth in the data collection efforts of companies across the world.
Right now, thousands of data-minded company leaders are investing in A.I. initiatives, and the appeals drawing them in makes sense. Enhanced A.I. driven computing performance, and the promise of being able to harness big data for deeper business analytics, are concepts that’ll capture the attention of any Ecommerce operation. But it’s important those operations don’t get ahead of themselves.
July 2024 Offer – For a Limited Time Only:
Get WordPress 99+ Plugins Mega Bundle for 15% off! Don’t miss out!
The Dilemma in a Nutshell
There is real potential in data science and machine learning, and it’s understood there’s hidden value behind all the data being extracted. But a lot of the excitement surrounding A.I. is causing premature expectations to form within some companies—and those expectations often lead to costly investments with little turnout in terms of business value.
To help avoid waste yourself, let’s look at the current state of data science and the reasons why we shouldn’t blow A.I. out of proportion.
Current Hurdles in A.I. & Data Science
According to an analysis by Gartner, 85% of data science projects end in failure.
That number may sound surprising at first, and perhaps even discouraging to businesses who’re considering their own data science initiative. But at the very least, after the deployment of so many ineffective (and the few successful) A.I. data systems, some traceable factors regarding whether a project will prove worthwhile have been discovered.
Isolated Data Analyses
Big data thrives when it has ample information behind it, but due to the protected intellectual property practices of countless companies, data scientists often don’t have access to most the relevant information they need.
Many projects have their analysts operating within independent company systems that can’t access the relative data analyses completed by others. But this poor coordination doesn’t stop at the general wealth of data science knowledge out in the world that’s trapped behind legal barriers. Many companies have disjointed data projects, with separate employees working independently on the same business goals, for no good reason.
This all creates a sincere problem with the data transparency A.I. systems and scientists require, resulting in low business returns.
Emotional Executive Decisions
Speaking of collaborative analysis, According to Gyro survey of over 720 business leaders highlights the dangerous nature of subjectivity in data science decisions.
To summarize, the report shows that as business decisions become more complex, the factors driving human judgment become less rational and analytically based. The more difficult the issue, the more emotional the deciding factors end up becoming.
A large reason for this gap is unpreparedness on the part of companies, leaving their teams to navigate unexpected data science challenges with little strategy or direction. They miss three core understandings what any AI projects require:
- Deep planning and evaluation
- High involvement of all parties- executives, data team, product managers, etc
- Continuous follow up by executive
This also brings us to the next, and perhaps one of the largest, problems with A.I. in data science.
Unskilled Implementation
Skill shortages in A.I. systems are a major issue for companies, especially smaller ones, who are trying to take advantage of data science.
The value of a Data technological background is rising,and more people are enrolling in A.I. education. But as it stands today, the lack of savvy data scientists is preventing many operations from receiving any guidance of real value. Of course, many under-prepared data science initiatives move forward regardless, lacking clear goals and almost always failing to integrate efficient analytical systems.
Yes, the call for data mining experts well-versed in A.I. is out there, and in upcoming years expertise in this area will surely spread. But the fact for now is, unless a company has the in-house data science knowhow, or a partner who does, they are setting themselves up for disappointment.
Additional Common AI Hurdles
- Unrealistic expectations from the executives, that think that AI can do anything, and solve any problem.
- Lack of awareness among executives as to the high costs of AI, imagining that the cost can be easily compared to any other software development.
- Because data is the core ingredient of any system, all and every change in organization data will cause any AI project to fails unless AI team is aware of changes in time.
Conclusion
So, there is a ton of appealing data out there just waiting to be used.
But in truth, a lot of companies don’t yet have the resources or strategies to successfully apply A.I. data science on their own. At the same time, the forecast around A.I. is being watched intently, we’re always learning more, and the above-mentioned problems are eventually solvable.
What’s most important right now is that businesses understand not only the potential of this budding data science technology, but its current limitations as well. By getting a grip on A.I. and moving forward with a realistic mindset and proper guidance, companies can save themselves from unrealistically high expectations and faulty investments.
More than that, by staying aware of these common pitfalls, businesses will be that much more capable of cultivating future data science initiatives that are actually effective.